test train split sklearn with shuffle in old package|shuffle split cross validation : agencies random_state will set a seed for reproducibility of the results, whereas shuffle sets whether the train and tests sets are made of from a shuffled array or not (if set to False, . Resultado da American Horror Story: Coven - Full Cast & Crew. 2013 -2014. 3 Seasons. FX. Drama, Horror, Suspense. TVMA. Watchlist. Where to Watch. A .
{plog:ftitle_list}
webAprende a ganar las recompensas diarias para desbloquear tus historias favoritas
The shuffle parameter is needed to prevent non-random assignment to to train and test set. With shuffle=True you split the data randomly. For example, say that you have balanced binary classification data . random_state will set a seed for reproducibility of the results, whereas shuffle sets whether the train and tests sets are made of from a shuffled array or not (if set to False, .Split arrays or matrices into random train and test subsets. Quick utility that wraps input validation, next(ShuffleSplit().split(X, y)) , and application to input data into a single call for .
You could just use sklearn.model_selection.train_test_split twice. First to split to train, test and then split train again into validation and train. Something like this: X_train, X_test, y_train, y_test. = train_test_split(X, y, test_size=0.2, .
train test split shuffling data
In this tutorial, you’ll learn how to split your Python dataset using Scikit-Learn’s train_test_split function. You’ll gain a strong understanding of the importance of splitting your data for machine learning to avoid underfitting or . In this tutorial, you’ll learn: Why you need to split your dataset in supervised machine learning. Which subsets of the dataset you need for an unbiased evaluation of your model. How to use train_test_split() to split your . In this tutorial, I’ll show you how to use the Sklearn train_test_split function to split machine learning data into a training set and test set. I’ll review what the function does, I’ll explain the syntax, and I’ll show an . Train Test Split Using Sklearn. The train_test_split () method is used to split our data into train and test sets. First, we need to divide our data into features (X) and labels (y). .
ShuffleSplit # class sklearn.model_selection.ShuffleSplit(n_splits=10, *, test_size=None, train_size=None, random_state=None) [source] # Random permutation cross-validator. Yields . It is used to split arrays or matrices into random train and test subsets. train_test_split(*arrays, test_size=None, train_size=None, random_state=None, .
random_state just sets the seed for the random number generator, which in this case, determines how train_test_split() shuffles the data.. Using random_state makes the results of our code reproducible.. .I know that train_test_split splits it randomly, but I need to know how to split it based on time. . you can try TimeSeriesSplit from scikit-learn package. So the main idea is this, suppose you have 10 points of data according to timestamp. . test = train_test_split(newdf, test_size=0.3, shuffle=False) Share. Improve this answer. Follow
Added in version 0.16: If the input is sparse, the output will be a scipy.sparse.csr_matrix.Else, output type is the same as the input type. Isn't that obvious? 42 is the Answer to the Ultimate Question of Life, the Universe, and Everything.. On a serious note, random_state simply sets a seed to the random generator, so that your train-test splits are always deterministic. If you don't set a seed, it is different each time. Relevant documentation:. random_state: int, RandomState instance or None, optional .
In this tutorial, you’ll learn how to split your Python dataset using Scikit-Learn’s train_test_split function. You’ll gain a strong understanding of the importance of splitting your data for machine learning to avoid underfitting or overfitting your models. . (X, y, test_size=0.3, random_state=100, shuffle=True) We can now compare the .Extension of @hh32's answer with preserved ratios. # Defines ratios, w.r.t. whole dataset. ratio_train = 0.8 ratio_val = 0.1 ratio_test = 0.1 # Produces test split. x_remaining, x_test, y_remaining, y_test = train_test_split( x, y, test_size=ratio_test) # Adjusts val ratio, w.r.t. remaining dataset. ratio_remaining = 1 - ratio_test ratio_val_adjusted = ratio_val / . conda upgrade scikit-learn pip uninstall scipy pip3 install scipy pip uninstall sklearn pip uninstall scikit-learn pip install sklearn Here is the code which yields the error: from sklearn.preprocessing import train_test_split X_train, X_test, y_train, y_test = train_test_split(X,y,test_size=0.2,random_state=0) And here is the error:
The Basics: Sklearn train_test_split. The train_test_split function is a powerful tool in Scikit-learn’s arsenal, primarily used to divide datasets into training and testing subsets. This function is part of the sklearn.model_selection module, which contains utilities for splitting data. But how does it work? Let’s dive in. from sklearn.model_selection import .
I know that using train_test_split from sklearn.cross_validation, one can divide the data in two sets (train and test). . label patches :param percentage: list of percentages for each value, example [0.9, 0.02, 0.08] to get 90% train, 2% val and 8% test. :param shuffle: Shuffle dataset before split. :return: tuple of two lists of size = len .The answer I can give is that stratifying preserves the proportion of how data is distributed in the target column - and depicts that same proportion of distribution in the train_test_split.Take for example, if the problem is a binary classification problem, and the target column is having the proportion of:. 80% = yes 20% = no Since there are 4 times more 'yes' than 'no' in the target . If I want a random train/test split, I use the sklearn helper function: In [1]: from sklearn.model_selection import train_test_split .: train_test_split([1,2,3,4,5,6]) .: Out[1]: [[1, 6, 4, 2], [5, 3]] What is the most concise way to get a non-shuffled train/test split, i.e. [[1,2,3,4], [5,6]] EDIT Currently I am using
Paso 4: use la clase dividida de prueba de tren para dividir los datos en conjuntos de prueba y entrenamiento: Aquí, la clase train_test_split() de sklearn.model_selection se usa para dividir nuestros datos en conjuntos de entrenamiento y prueba donde las variables de características se proporcionan como entrada en el método. test_size determina la parte de .class sklearn.model_selection. StratifiedShuffleSplit (n_splits = 10, *, test_size = None, train_size = None, random_state = None) [source] # Stratified ShuffleSplit cross-validator. Provides train/test indices to split data in train/test sets. This cross-validation object is a merge of StratifiedKFold and ShuffleSplit, which returns stratified .Added in version 0.16: If the input is sparse, the output will be a scipy.sparse.csr_matrix.Else, output type is the same as the input type.New in version 0.16: If the input is sparse, the output will be a scipy.sparse.csr_matrix.Else, output type is the same as the input type.
Although Christian's suggestion is correct, technically train_test_split should give you stratified results by using the stratify param. So you could do: X_train, X_test, y_train, y_test = cross_validation.train_test_split(Data, Target, test_size=0.3, random_state=0, stratify=Target) The trick here is that it starts from version 0.17 in sklearn.
版本 0.16 中的新增功能:如果输入稀疏,则输出将为 scipy.sparse.csr_matrix 。 否则,输出类型与输入类型相同。
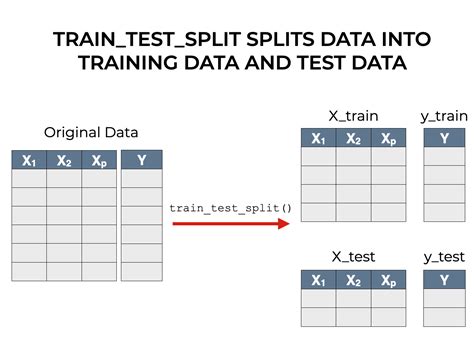
from sklearn.model_selection import train_test_split . There are a couple of arguments we can set while working with this method - and the default is very sensible and performs a 75/25 split. In practice, all of Scikit-Learn's default values are fairly reasonable and set to serve well for most tasks. However, it's worth noting what these defaults are, in the . Step #1. We’re going to use a couple of libraries in this article: pandas to read the file that contains the dataset, sklearn.model_selection to split the training and testing dataset, and .Added in version 0.16: If the input is sparse, the output will be a scipy.sparse.csr_matrix.Else, output type is the same as the input type.
unity test package
unity test framework package
Parameters: *arrays: sequence of indexables with same length / shape[0]. Allowed inputs are lists, numpy arrays, scipy-sparse matrices or pandas dataframes. test_size: float, int or None, optional (default=0.25). If float, should be between 0.0 and 1.0 and represent the proportion of the dataset to include in the test split. Now that we are familiar with the train-test split model evaluation procedure, let’s look at how we can use this procedure in Python. Train-Test Split Procedure in Scikit-Learn. The scikit-learn Python machine learning library provides an implementation of the train-test split evaluation procedure via the train_test_split() function.
New in version 0.16: If the input is sparse, the output will be a scipy.sparse.csr_matrix.Else, output type is the same as the input type.
How to use sklearn train_test_split to stratify data for multi-label classification? . I would use instead use the following function that uses the iterative-stratification package. This only requires 2 seconds on the same data: . stratify=None, shuffle=shuffle) assert shuffle, "Stratified train/test split is not implemented for shuffle . In Python, train_test_split is a function in the model_selection module of the popular machine learning library scikit-learn.This function is used to perform the train test split procedures, which splits a dataset into two subsets: a training set and a test set. train_test_split(*arrays, test_size=None, train_size=None, random_state=None, .
If float, should be between 0.0 and 1.0 and represent the proportion of the dataset to include in the test split. If int, represents the absolute number of test samples. Stratified Train/Test-split in scikit-learn using an attribute. Related. 2. Using StratifiedShuffleSplit with sparse matrix. 2. Sklearn StratifiedShuffleSplit with pandas. 4. Stratified GroupShuffleSplit in Scikit-learn. 2. The StratifiedShuffleSplit (in sklearn) returns different proportion each time. 1.
train test split shuffle false
train test split documentation
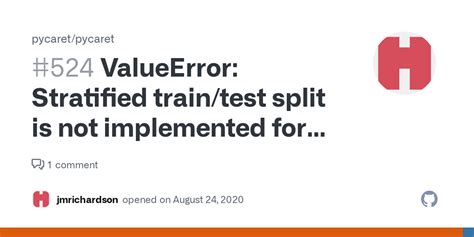
1 dia atrás · Updated: February 29, 2024: We found the latest Coin Master free spins .
test train split sklearn with shuffle in old package|shuffle split cross validation